How AI can change accounting: Agents & LangGraph
Download MP3Alrighty, awesome. Well, welcome everybody to episode 20 of the Break Even Brothers podcast. It's pretty, I have to say, it's pretty impressive, Brad. I'm giving ourselves a pat on the back. Yeah, pretty epic. Big two-zero. We have some merch. I know we talked about it in a different episode, but I'll have to showcase merch maybe for episode 21. But it's something that I think our viewers will be very, very excited about—a one-of-a-kind, limited item, but I don't want to speak too much on it right now. Nice way to sell it. Awesome. Well, it's the day after a nice holiday weekend. Did you do anything fun over the weekend?
Nothing crazy. Watched a movie, did some cleaning. Honestly, a pretty chill weekend. I got a lot of work done for Split My Expenses, which I've been dying to do. I think I even said the same thing last podcast episode. I'm building up the, the, the kind of momentum of getting the mobile app out there. I, you know, I told myself, end of this first half of the year, and if you look at the calendar, it's coming around very quick. So, uh, did some fun life stuff, but outside of that, I think I'm pretty happy to report that I've made significant progress on the mobile app. And I won't go too deeply in the pod, but working on some of the data syncing side, which is how the iPhone app keeps up to date with the server-side data source. So, uh, there's a whole bunch of technologies out there for it. Super complicated. But yeah, I'll stop there. How's your weekend?
No, it's good. Yeah, that's cool to hear. Um, yeah, good weekend. Worked a bunch too, but in a fun way, which I'm super excited to talk about because I was working on stuff for the—not for the podcast, I was working on stuff that would be fun to talk about on the podcast. So coming into this one with a lot of energy and yeah, ready to roll. I think we'll have a good episode this one. So yeah, it's been a whirlwind. I feel like these past two weeks have shown us that things don't stop in the AI race. It's only getting better and better, and it's exciting being, you know, kind of in this space today, and even the past six months, I think it's just super awesome to see what companies are putting out there. And, uh, you know, like we say, it's just going to get better from here. So yeah, super excited.
Yeah, totally. Well, I think we wanted to kick this episode off with a fun little challenge of a blind ranking of AI apps. So why don't you tell us a little bit more about how we're going to do that?
Yeah, so I've seen this trend on TikTok. It's not necessarily used for AI things, but I wanted to apply it to our podcast. So basically, it's blind ranking. So you don't know, you know, the kind of the category of the list, but you don't know what's exactly in the list that you're guessing. So today, we're going to do a blind ranking of our 10 AI chat apps. So I've asked AI to essentially come up with a list of 10 of these options. I've handed it off to our editor, Ellen, in the back room, and she is going to present us a current chat app. And Ben and I are going to have to work together to place it. So one being the best AI chat app and 10 being the worst. Again, we don't know what's coming, so we'll need to work together to kind of place these. And yeah, we'll see what we end up with and we'll share it. So let's kick it off with that. Ellen has put it into our chat: Perplexity AI.
AI. So Ben, what do you think? I've seen it a bunch. I haven't used it myself. I see it usually on X, people will be like, "Ask Perplexity," and explain it.
Yeah, so I'm gonna give it, I'm gonna give it a seven. I haven't used it, but I'm just going off of, uh, okay, where I've seen it.
Okay, that's fair. I also agree. I see it on Twitter. People tag their Perplexity, like, AI bot, and it basically will just take a look at the tweet, kind of explain it or summarize it or whatnot. I think it used to be pretty good from what I've heard. Now that other AI chat apps have integrated web search in the past two months, I think it's definitely lower on the list. So I would also go five—probably not terrible. So I'm okay doing seven or eight. Let's book it for seven, I think.
Okay. Seven it is. All right. Let's see what we got next. Replica. Okay. I'm not sure I've heard of this one. Have you heard of this?
No, I haven't. So, okay, just to be fair, we asked AI to come up with an AI list, and this is using, uh, a GPT O3 model with web search. So this is probably a valid AI chat app. However, Ben and I have no clue what this is. So for that reason alone, let's go with number 10. What do you think?
Number 10? I was gonna go number nine, just to save it for maybe one that I know sucks.
Yeah, okay. Let's go nine. I don't know what that is.
Okay, read that one out for us.
Uh, Gemini. So obviously Google's product. I'm feeling like it's number one or two for me right now.
Ooh, hot take. Yeah, it's actually really good. They made it a lot better. I think they came out with it quite a while ago, but over the past few months, the Gemini team has invested more resources into AI Studio, and it's a really good interface that pastes in a ton of text. It takes in audio. It takes in files. It integrates with Google Drive. Huge win. You can do big files. Honestly, it's definitely top three, if not top two for me as well. I do feel, number one, it's hard to give up. So I'm probably going to slot it for two. I imagine ChatGPT is on the list, which could be a top contender as well. You know, I think that's likely where mine will end up. But what do you think? Two, one, three?
Well, I'm including, like, all of Google's AI, like, products in this because they have something called NotebookLM, which I think you and I have exchanged texts about, which is super cool. I've been playing with that a bunch lately. So for that reason, I would go number one. But number two, I understand two. So I'm not gung-ho on either one.
I think I should put a slight caveat here that I think AI Studio is very geared towards developers. It's a power tool for developers and people who are very tech-savvy, where I think something like a chat AI app should probably be a little bit more dumbed down but powerful. So for that, I'd probably lean towards two because I assume ChatGPT is in the list and it's likely headed in that direction. So if you're okay with it, I'm going to slot it with two.
Okay. Yeah, let's do it. A solid, solid product from Google. Honestly, it's a killer. Okay. Interesting. So we got Copilot from Microsoft. And I think, I don't know if I classify it as a chat AI app. I think Copilot is a very muddied name in the Microsoft product space. It's GitHub Copilot, kind of the editing experience in the IDE. There's Copilot built into the Windows OS, I think, which is kind of like a Siri or something. And I don't know, I think there's a Copilot on Bing search too. Do you know which one this might be referring to?
Yeah, I read that as the GitHub version, but then also as, like, the one that comes with your Microsoft 365 subscription, which is just, it's just bad. Like, in my experience, it's pretty horrible. So I was thinking eight. I was thinking eight on that one.
Okay. Yeah. Yeah, let's slot it at eight because I've also seen screenshots online of people asking it to do rudimentary things and it just cannot compute. And it's something that's built directly into the product. So I think eight is reasonable for if you're going to integrate an AI model into, like, an AI chat and it can't operate on things that its core product is for, it's probably not very good.
Well, and I would just add one thing with that, too. You know, a lot of times companies are either like a Google Workspace or they're Microsoft. And in my experience, it's been a lot of Microsoft, you know, Windows, Excel, all that fun stuff. And so they had a, I feel like they have a ton of potential, but they just have not been able to, like, translate that into—whereas, like, Google Workspace is killing it. Like, again, Gemini is amazing. NotebookLM is really cool. Google Docs, Google Sheets. It's all kind of, I'm seeing it more and more. Yeah. And like they have a much better AI kind of built into that system. So, like, Microsoft could have done that, but so far it's not been good. So yeah, just my experience.
Cool. And then next up we got Grok. So this is Twitter's—or XAI, as they call it—their chat AI app. And this one, honestly, it's pretty good. It's in my top five for sure, for me personally. It has really good web search. So if I ask it to do something like trip planning, it will scrape like 60 or 80 web pages for me, have a nice summary. It's very DeepSeek-like. So if you use DeepSeek chat, it kind of feels similar. It has good thinking tokens. I think it doesn't handle files, or at least I haven't tried that. So I think that might be a slight knock, but it does have the Twitter data source, kind of like fetching tweets and doing all that. It's kind of an underpinning data source that other companies find a little bit harder to access.
Yeah, I haven't used it at all. So I have to kind of recuse myself from that. The only thing, my only, like, bias, I guess, is that, like, Twitter can be such a cesspool sometimes that, like, you know, I would kind of just shy away from it just for that alone. But again, haven't used it. Sounds like it's pretty good. I'm happy wherever you want to put it.
Okay. So I will place it then. I think since I go to it and I use it, that is a strong indicator that it's a part of my arsenal. Where it exactly fits... we have, you know, three through six open. I think I'm gonna place it at four. And I think because I can, you know, I'm thinking what's coming, I'm thinking that's a good spot for it. So I'm gonna slot it as four. Solid app. If you haven't used it, definitely try it out. It's impressive.
Oh, Quora... Poe—or Poe, sorry, Poe—which I guess is built by Quora. It's a shame that nine and ten are taken, because I feel like that's where it belongs. I'm saving number nine for one. I hope it's on there, though. That's my thought.
Yeah. I never used it. Have you used it?
I have not. I mean, I've heard about it. It came out very early in the AI lifecycle, so I'm not sure if they still actively push it or what its status is. No one that I know uses it or talks about it. So yeah, 10 is open, but yeah, I don't know. I mean, anything past five is not great. So we have five, six, and ten so far reserved. I would... yeah, I mean, it feels wrong to put it above Perplexity because, like, I've seen that used and, like, it seems like it's decent. But man, there's, there's one at 10... or if it comes up, I'm gonna be upset... that we gave nine away. But I feel like for that reason, just by, you know, the way this game is played, I think we have to do 10. It can't be better than Perplexity.
I'm not understanding what your 10 might be. And don't tell me because I'm curious. But let's roll 10 with this because, I mean, Replica should probably be 10 at this point. But we didn't know what was coming, which is the fun of the game.
Yeah. Pi. I've never heard of this one either.
I've also not heard of this one. It's Pi from Inflection. So I think when we had talked about chat AI apps, maybe this is getting a little bit of a reach. And maybe this exists, but maybe not a ton of people use it. Sadly, we have filled up our back half almost at this point. So I think by default, since we don't know it, we don't use it, it's got to slot into six, which hurts a little bit because I've heard of Copilot and Perplexity, which are slotted at seven and eight. So what do you think about six?
That's got to be six. Yeah. Yeah. I hate to do it. Okay.
Okay. Big hitter. Big hitter. ChatGPT, OpenAI, first to market. Some would say the best macOS app out there, iPhone app as well, first-class experience. Um, I'll just stop there. What do you think?
Yeah, I think just for ease of use and options and, like, all things it can do, I'm going number one. To me, it could have been Gemini or ChatGPT for number one, but I think ChatGPT, I mean, it has all the different models. It's got... if we're just talking about OpenAI in general, I guess this is specific to chat apps, but yeah, it's just, it's got the most, um, widespread adoption, ease of use, different levels of models depending on what you're looking for. Deep Research is amazing in my experience. Voice mode as well. They've got some cool features out there. I think it's easy. We're biased. ChatGPT, if you're not using it as your chat AI app, please get with the times and download and try it out. Yep. Okay. And I guess Chat Teams, I think we've mentioned this before, but Chat Teams does not train on your data, which is great for enterprise use. Yeah, they've definitely changed that.
Cool. And then we have Claude from Anthropic. So another incredible tool. I was expecting to slot this in my top three, and I... I luckily... number three is open in our list. Yep. I... yeah, that's an easy placement for me, but I'll let you comment on that.
Yeah, number three. I think that the top three, I think is perfect. I think wouldn't touch a thing. Yeah, good, good job us. Good job. Yeah, Claude is pretty solid. MCP stuff is really cool. I don't use it much anymore, but like, it seems like it's really good for, like, what it... like the MCP thing at least is kind of my last kind of dabbling in it and, um, yeah, it's pretty good.
Yeah, one last question before we get to the final chat app. Any guesses for what number five might be before we get it revealed? Well, number five. Okay, yeah, sorry. Yeah, the last one on the list. No, no, I have no idea because I don't have it either. I don't know. Yeah. Yeah, I'm not sure. Ellen, hit us with the last one.
Yeah. Character.ai. Never heard of it.
Okay. I've heard of it. It had a huge market share, I think maybe middle of last year, late last year. They were like neck and neck, I think, with ChatGPT because people wanted to talk to AI apps. It's kind of like Character.ai, the product was you could talk to AI agents that had personas and profiles. And it was just kind of having a friend online virtually. So I think it is a chat AI app. I think we were thinking more productivity-focused; this is more entertainment category. Like, given we only have number five, it's gonna slot as number five. And if we take a look at the list, I think we didn't do too bad. So I slot that in at number five. And then maybe you can give us the rundown of what you think and, you know, what our final list looks like.
Yeah, I think one through three, and one through four. Again, I've only heard good things about Grok. I just haven't used it. So I think one through four is pretty solid. You know, honestly, I think Perplexity is the only one that's probably out of place, in my opinion. That should probably be five. Then the rest, I think, is pretty good. The one that I thought was coming out... I guess if we go top to bottom: ChatGPT, Gemini, Claude, XAI or Grok, Character.ai, Pi by Inflection (Pi like the math Pi, not, you know, apple pie), Perplexity AI, Copilot, Replica, and Poe by Quora. So that's our final 10. And yeah, I think the only one that's really out of order there is probably Perplexity, and the rest can, you know, pretty much stay the same. Yeah, the one that the one was to say... the one that I thought was... I guess it's not chat, but I was just going to just poo-poo on Apple Intelligence because that just seems like it's just such a lost opportunity. And I don't know if they're going to be able to recover or catch up from how poor that is right now.
Yeah. I'm surprised DeepSeek didn't make the list, but I'd probably put that on there, and then maybe Apple as well. So interesting to see what the O3 model came up with as a chat AI app. And I was the one who prompted it. And as we all know, prompts do matter. So maybe I can make a better one next time. But that was awesome. I think we did decent. I think top three, we nailed it. Uh, you know, four to six, it's okay. Then there were definitely some unheard-of ones. So that was fun.
Yeah, yeah, that's cool. Cool. Alright, well, it has been a jam-packed two weeks. Last time we recorded, I think, was early April, roughly two weeks ago. And since then, there's been a ton of releases. So I had just kind of opened up a little notepad for myself and wrote down a few of these from the kind of frontier AI model space. So just going down the list, we have OpenAI released two major releases probably the past two weeks. One is their GPT-4.1 models. And the unique thing about their 4.1 models is actually API-only. So they're not releasing it on the ChatGPT app. And it's basically trained to use tools. And the trend of building agents and MCP servers and tools is that all these AI models were not previously trained to use tools. They were kind of retrofitted to use them after. Supposedly, GPT-4.1 and their other newer models are more equipped with training data sets that use tools, so they can kind of describe how to do that. So they came out with GPT-4.1, 4.1 mini, and 4.1 nano. And I think that is a kind of direct replacement to GPT-4.0, which has like 4.0 and 4.0 mini. And then their second two-parter release was O3 and O4 mini. And probably what you're thinking right now is, damn, it's hard to keep up with, you know, which is what, and O2, O3, O5. And I'm with you. It's very complicated. I'd take a look at their models page multiple times, and I had to kind of put this together. But to kind of break it down: O3 is a very intelligent large model, O4 is pretty smart, but it's the mini version, so O4 mini. And these are all available on the API today. And I think O3 and O4 mini are available in the chat. And again, these are very good at tool-calling. They have web search now. So it's a pretty solid offering from OpenAI. Super exciting.
And then, yeah, so next step, Gemini: so we have 2.5 Flash. Google's been really killing it, as we've talked about in previous podcast episodes. If OpenAI releases something, Google releases the next day. If Google releases something, OpenAI comes the next day. So we had 2.5 Flash, and this is kind of the introduction of the thinking budget. So Google allows you to say, how much do you want this model to think? Previously, models were just, you know, you asked a question, it responded. Now it has this internal thinking mechanism to get a higher quality response. And Google's allowing us to change that on a per-request or per-chat basis. And Google, you know, kind of continuously wins this price-per-performance bucket with a generous free tier. So they're doing well to adopt AI for the masses, for engineers. And will they keep us? Will their price-per-performance remain top-notch? Who knows? We'll see.
And then we also have releases from Twitter. So like XAI, like we talked about earlier, they finally released Grok 3 and Grok 3 Mini, which power the Grok AI chat. Pretty similar to DeepSeek models. So again, like I talked about, they're really good at web search and good at thinking. And I don't use them on the API yet. And they're not, I think they're in Cursor and other AI IDEs, but definitely the OpenAI and Google Gemini models are what people are using today.
I think the big trend we're seeing is that benchmarks aren't becoming that useful anymore, which is a really weird thought. People get very tied up on, 'Oh, there's a new release. How does it compare in the benchmarks? What are the raw numbers?' We got 50 different benchmarks. Sounds useful, but I think at the end of the day, people are getting much more tied to 'vibes,' which also sounds stupid, but it's very much a thing. And if you've kind of 'vibe coded' before, I think you'll really understand it. But you can have a really good model on paper, but how well does it follow your instructions? How well does it understand you as a human? You know, when we're writing prompts in some of these AI IDEs, they're not very pretty—typos, et cetera. And I think on these benchmarks, it's very structured, rigorous, and it's just a different environment. So I think the new trend is kind of emerging that benchmarks don't tell the full story. You can have a new model that comes out that's good, but not great on the benchmarks. It still performs well, which six months ago would sound like a completely backwards thought. So very interesting, huge releases, lots of stuff available in the API. And if you haven't tinkered with these in Cursor, if you're using Cursor or Windsor, definitely try them out. It's very much what works for your use case. But I think Google I/O is like in a few weeks, there's going to be new stuff coming. I'm really excited to see this heat up. I think these past two weeks have been a real inflection point of extreme competition.
Yeah, the pace has been crazy. And, you know, I think probably a month ago, I was using, you know, ChatGPT pretty exclusively. And now for this last month, I've been using Gemini pretty much exclusively. Um, yeah, also for the chat, but also, um, in Cursor, you know, doing some coding, um, which has some stuff to update or share with this podcast, but, um, all been in—or all been using, you know, the Gemini 2.5 Pro, I think is the one I'm using in Cursor. Yeah, and it's, it's pretty remarkable. And I think one thing that, um, it just takes time, and I think once you—people that have used different models a lot can see it—like, they kind of respond in different ways. Like, they're both maybe right on how to do something, but like, Claude might answer something a different way than, like, how Gemini does. And it's, it's really nuanced. Like, I couldn't point out specifically what it is. But like, I did some stuff, and I was asking Gemini, and for some reason Gemini kept timing out in Cursor. And so I just switched to Claude, and it gave me, like, still a right answer on something, but it was, like, just formatted differently. The response was just, you know, the vibe was just different. You know, what can you say? It was just different. So, but yeah, I mean, they're, they're incredible, just the how good they are at what they do, because you can get so much done.
The cool thing is, if you have code written for OpenAI, lots of these other AI providers like XAI or Google Gemini, they are, quote-unquote, 'OpenAI API compatible,' which is a mouthful. But what that means is basically when you use a library, it's making API requests to OpenAI servers. When Google is OpenAI API compatible, it means you can change the URL in which you're sending these OpenAI chat requests to a Google domain. You don't have to change anything else of your code, but they return the same response format that OpenAI does so that you can switch to them easier. So I had spent a lot of effort pulling in Google Gemini libraries in PHP using them for Split My Expenses. But then I realized I could rip out all of Google's extra code, literally change maybe two or three lines to configure my OpenAI library to point at Google's domain instead of OpenAI's domain, and change the API key to be the Google API key. So it feels like it's OpenAI, but it's Google under the hood. And that makes it 10 times easier to actually make these requests and iterate on different models. So I'm with you. I think 2.5 Flash and 2.5 Pro are excellent, incredible models, top of the leaderboard, and so you really would be unwise to use something different at the moment. Each use case has different kinds of constraints, but right now, general purpose, you know, latency, price-per-token, Google is killing it. And I'm excited to see them kind of continue to do well. I think they've spent a lot of effort to make it better, and I'm sensing it's only going to continue from here.
Yeah, and I think especially for folks that are using, like, if they work at a place that uses Google Workspace, like dig into those tools. And again, I think we should talk about NotebookLM a little bit in a separate podcast, but super cool tool, like really fun, especially for, like, non-technical people. It's more of, like, a knowledge base kind of thing. But, um, yeah, it is, we'll, we'll talk about that in a different podcast episode because I could probably go pretty deep into how fun that's been. Um, actually it's, it's actually one of my bookmarks. It'll touch on a little bit in my bookmarks actually. Um, but we'll save that for the end. What I wanted to chat a little bit about with this podcast episode was, and what I was working on, you know, last few days, including over the weekend a little bit, was LangGraph, because I think this is actually a pretty significant library/framework for, I'd say, accountants to know. And what I kind of mean by that, too, is I think this is also recognizing that I think the role of an accountant—and not necessarily public accounting, where, like, you have clients and you're working on your clients' books, but like, people that are working at a company doing the accounting for that company—I think that role is going to change. I think it's going to happen pretty fast. You know, accounting tends to move a little bit slower because we've got to be careful. There's controls. There's, you know, you've got to be accurate. So get all that. But, you know, the era and the time of doing things manually, just like the process of doing things, that's got to get changed a little bit. And so I think LangGraph is a really cool framework to kind of accomplish that.
Are you telling me that the accounting landscape is going to change? This sounds like some fear-mongering, I think.
Well, I don't think it's fear-mongering. I think it's a little bit of reality. You know, I'm not, you know, it reminds me of there was that story that the longshoremen were striking because, you know, there was some automation that was going to take place that they're already doing in China. But, you know, there's a union, the longshoremen, and all that to say, like, you can't fight technology. Like, it's coming, you know? So, like, all you're doing is delaying the inevitable. But LangGraph, I think we're going to touch on it here, it's not replacing accountants at all, but I think it's changing the skill set of what's important. I think... because I've done and been a part of automations, you know, pretty much all throughout my career. And, um, you know, the way that they've been done in the past has had a lot of limitations, and so LangGraph, I think, solves a lot of those limitations, plus also things like, you know, chat, being able to have non-coders be able to kind of get there a little bit more on their own—not fully, but get there a little bit more on their own. So what LangGraph is, for those that don't know, it's basically a library or a framework. There's a Python library, I think there's also a JavaScript one, but I'm, I've been using Python. And basically, it really helps you kind of nicely outline a complicated process or a complex series of steps. And I think a lot of times for accountants and for accounting, you know, tasks that you might have, you're doing kind of multi-step analysis or multi-step, you know, data manipulation to get a report into a journal entry or to get a report and run it through a series of checks. And what's a limiting factor with generative AI is that, like, you can't have guesses in accounting. It's all, it's all dollars and cents, right? But what's nice about LangGraph is it's, you can route to, like, paths, like specific paths for code to run. So you're not necessarily having AI do an analysis for you, but you can code. This is what the steps are. And so that was a lot, but I think that's the first point to kind of hone in on is that you are not having generative AI, like, analyze something, or at least that's not my kind of first use case with this. But you can basically encode, outline the series of steps with LangChain that will run, and that's always in a, you know, it's a fixed process. It's not, you know, generative AI doing its hallucinating, right? It's, you know, this is how I do my steps. It's A, B, C, D, E. And, um, LangGraph just provides a nice way to kind of put those things together.
I've used LangChain when it came out a long, long time ago. And when I look up LangGraph, which is a weird word, it looks like it's under the LangChain domain. So is LangGraph more the agent side of LangChain?
Yeah, so LangGraph, as I understand it, you actually don't have to use LangChain at all. You can use LangGraph, like, directly with, you know, the agent SDKs from OpenAI or the other ones. But what LangGraph really is, it's a way to kind of—at least this is kind of how I've adopted it for accounting—is it's a way to kind of break up a series of steps that you might be doing and kind of chain them together. So kind of, you know, kind of similar to LangChain, but they basically define nodes. So you think of it as, like, steps. You define those nodes, and then LangGraph kind of lets you put those together nicely where it's like, 'Hey, this node goes to this node, and then that node goes to this node, and then it ends here.' And so the specific kind of process that I was working on over the weekend was basically, like, a financial statement analysis where every month I have a person that, like, runs a report and they run a series of checks to make sure, you know, 'Hey, this makes sense. We are clear on these numbers here, blah, blah, blah.' And then spits out an output that says, 'Hey, thumbs up, we're good' or 'thumbs down, we're not good.' And that's every single month. It's a consistent thing. All we're doing is changing the month that we're doing analysis for. And so what I was able to do was define, like, what are the steps in that process? Basically code that each step was a node, an individual node. So getting the financial statement data, that's a node. I used Pandas, which, you know, you've probably heard us talk about on this podcast before. It's like a data analysis library in Python, can read Excel files beautifully. And so, you know, get the financial statement data, had a Pandas data frame. So that's hard—like, that's not, I shouldn't say hard-coded, but that is not AI. That is just pure programming that's going and grabbing that data. Right. So there's no hallucination. You're just grabbing the data. So that was the first node. The second node was, okay, what are our requirements? Like, what does this financial data need to look like? And so you'd code the second nodes. Basically, I think I have, like, four or five nodes that I made that make up this process that this individual was doing, and then you can kind of chain them together. And the second part that's really nice about LangGraph is state management, which state management in the context of web development drove me crazy. That was always my frustration with JavaScript libraries. Yeah, but really it's basically, at least in the sense of LangGraph, it's like a persistent layer of data that goes all through your different nodes. So basically, as an example, and not to get too technical here, but like, the person when they ran this analysis, they needed to have the month, like, what month are we running this for? You know, March and February. Yeah. And basically that was, like, part of the state. So every single time they go through that step or go through those nodes, that node can access things in the state. So it's like, okay, we are doing... if we say March, you know, node B or sorry, node A can say, okay, we're doing this as of March. And then when you get to node B, it can go access that state that you defined. And so it kind of like, you can kind of build this little brain, as, like, state, and access it in the different nodes. And so that's something that's really helpful, too, because a lot of times in programming, at least in my experience with Python, it's like, you know, you define these functions, and then some of the variables in those functions are only available in those functions. Otherwise, you have to, like, return them and call them. And that can be kind of tricky for non-programmers. But like, having state where you're like, 'Hey, these are all the things I need to know as I do this process. I need to know the month. I need to have, you know, what's my, you know, if you have like a financial data, like what's my financial data, you know, what's my...' You can kind of outline all these different things that you need to know and make up that state. And then as you go through your different nodes, you can kind of add to that state or change that state, which is pretty cool. So those two things.
How similar was it to, like, MCP agents? Because I'm hearing, like, individual nodes. I'm thinking MCP server with tools, or is this a different approach?
Yeah, well, that's definitely where I'm kind of going with it. So basically, you have this programmed-out workflow that, you run it 100 times, you can get the same answer 100 times. There's no AI involvement, right? But where I wanted to kind of take this was making this process that I outlined, you know, with LangGraph, a tool for an AI agent. So basically I can tell the agent, 'Hey, run that process for me,' and it'll know, you know, based on the way that you define tools. And I was using LangChain for the tooling. You don't have to. Again, you could just use whatever SDK you want. But I was telling it like, 'OK, hey, I want to run this for March.' And basically the only involvement with the agent is kind of trying to understand what are you trying to do? And I only have one tool for this agent, so it's pretty easy. But obviously, as you get more and more tools, you have to be more aware of, like, you know, making sure your agent gets the right tool. Right. But then basically also kind of parses out what you're looking for. So you could say, 'Oh, can you tell me what it was last month?' And it kind of knows, 'OK.' 'Could you tell me what it was this month last year?' And it kind of knows, 'OK, this month is April, you know, but last year is 2023.' It does, like, the input analysis into feeding into the nodes. That's like the human interface and chat interface to be able to call these things.
Yeah. And like, that's super important in my experience because a lot of the times automation fails. It doesn't get adopted because people have a hard time running it on their computers. It's not, again, for non-technical people, we don't have GitHub. We don't have GitLab. We can't pull a repo down and run it. Most people don't know how to do that. It's just not part of the skill set that they were hired on for. But if you can make an agent that you can access in Slack, then you can just Slack that agent and say, 'Hey, can you run that for me?' And then, boom, it'll run that process, which I think is a much more approachable way for non-technical people to still be able to get to, like, custom workflows and custom automation. Because no matter how good software is, there'll be no software that can satisfy every single process that you do. And so I think there's still lots of room for people being able to make their own automations because every company might do something slightly different because they feel like they have to or because they feel like it's important. But you shouldn't have to throw away any hope of automation on that. You should be able to write these things in code. Again, Python, any language, really. But, you know, Python is so approachable now. So many great libraries. And you can use, you know, LLMs to, like, write the code. You know, I, again, I'm not a programmer, but I got this thing fully working in a matter of, like, two or three days. And it's pretty, like, it's pretty complicated steps. Like, there's a lot of steps involved. I think it's like 600 lines of code. And most of it is just the actual outlining of what the steps are. The agent piece of it is, like, I don't know, 60, 60 lines of code. But then basically, yeah. So having that LangGraph workflow be exposed as a tool to my agent. And then now the agent can run this process based on my input, which is...
So was it an official MCP server, or was the agent and tool that you described, like, built into their framework?
So it was... I built the tool, like the actual LangGraph tool, and then exposed it as a tool. So LangChain has, like, a decorator you can put on that says, 'Hey, this function is a tool.' You know, I don't know how it works under the hood. And then in your main program, when you make the agent, if LangChain does ChatOpenAI as a class, and then you define the model, you define the temperature, all that kind of stuff, then you can define tools. You can have it equal to the tools that you import from your main code base that you did all the logic in.
Okay, so maybe it's not traditional official MCP server and MCP tool, but it functions the same without the label, it almost sounds like.
Yeah, it's, yeah, it's not MCP specifically, but it's exposing an agent to a tool that, like, I made, you know? And so now this agent just has one tool, but you can also ask the agent, 'What's the weather like today?' and it'll respond to you. So, you know, it's a true agent. You know, it's not just, you know, me hitting, you know, `python main.py` on my computer, which doesn't really do anybody any good. But if you can port it and make it accessible, you know, now we're, now we're cooking with gas a little bit. And so, yeah, I think there's a lot of opportunity in accounting for that because there's so many things that we have just steps involved, you know? It's like, I can't have a direct connection from system A to system B to import things. So I got to do this manually. Well, it's like, you can do it manually, but you can code that manual process and then maybe make it a tool so that way, yeah, it's still manually being extracted, and then manipulated, then imported, but at least you kind of can get rid of the heavy lifting that comes with just monkeying with the data, which sadly is a lot of people's jobs.
Yeah, we're a big fan of automation. I think any process that takes data from one system, compares it with another, you know, import/output. It's all fun and it's all things that, like, hey, you're going to do it, but how do you make it a little bit easier to work with? How can you share that with people? Because I even worked on my Personal Capital MCP server this weekend. And as we talked last time, I was running into literal AI output-length limits. So Claude could only write 8,000 tokens. And part of those tokens was it chugging through my expenses, trying to literally write the expenses into the JavaScript code that I was trying to execute. And then I tried doing SQL. It kind of worked, but it didn't really work. And I went back to the drawing board, and I actually changed my MCP tool to not return JSON, but to return a CSV. Because I personally thought the output tokens that the LLM is looking at for JSON is very descriptive. So for every expense I had, there was a description, amount, and date. But on the next row, the same thing: description, amount, date. I could have less tokens and have AI parse it easier if I just had a header, and then under that was just a bunch of rows. And CSV is nice because if you don't have a value, it's just a comma. So no space, really. So I changed that. My tool is a lot better, which is cool. And I think when you're talking about the agent having the input, like choosing the month, I also found that to be extremely successful because I could say things like, 'What did I spend last month?' You know, I'm not typing in, like, March 1st, March 31st. It just knows based on the current day... that it, like, equips you with so much general knowledge... that that's how piecing all these things together makes it extremely valuable. Like, you can create this thing in LangGraph and you can choose the AI model. So, like, today we have fantastic models like O3, super high intelligence, you know, Gemini 2.5 Pro, super high intelligence. Next month, we could have something with, you know, in theory, two times the intelligence—probably not, but, you know, you get the point. And your same workflow exists, but now your agent knows how to do things better, would know how to converse better with you. Like, it's pretty incredible that you can build these building blocks, and then while you're building these things, the entire system gets better. You can change one line of your code, adopt a smarter agent, and now you have a reusable workflow with just increased knowledge. And I think that part is really, really exciting. And when we're building these flows, if it works in today's model, it's going to work very, very well in tomorrow's model. And you get the picture. The more we can bring to automations, the more real work we can do, because yes, it's important work. I think automation is always important work. But if you free yourself up from some of this mundane work, then you can focus on the bigger picture, you know, have more automations running for you. Just like when I see these AI IDEs, I don't see it as the end of programming for me. To me, it's like, I'm going to write programs that'll write programs for me. And then I just, you know, up the ladder I go, and who knows what I end up building? Like, it's such a stepping stone and multiplier that I think people, if you're excited about it, it gets you energized and you build stuff for yourself. And then you just kind of realize what's out there. It's a very kind of self-fulfilling loop.
Yeah, for sure. And I think, you know, the need for programming skills in particular is still relevant because as I was working on this... this automation, you have to be able to tell it, like, when to stop. You know, I think I mentioned before there was, like, a Ben Affleck quote where he was, like, someone asked if he was worried about AI taking over the movie-producing industry, and he was like, 'No, because AI doesn't know when something is, like, done, when to say I'm finished, like, this is perfect,' because it'll endlessly try to optimize and endlessly try to add features. And so you kind of have to know, like, enough to kind of get the job done. You know, a lot of times, you know, I used—of course, I was using Gemini—to, like, help write the code and do all, like, the basic stuff. But, like, a lot of the actual logic and, like, figuring out, you know, the actual code to write was myself, right? Because I know, as an example, you know, a lot of times your general ledger will have account numbers. And, like, those account numbers kind of tell you what it is. So, like, you know, account number 1000 typically is, like, cash. That's just kind of basic. And so, you know, you can kind of... you have to know, 'OK, if my cash accounts are like 1000 through 1000... or 1500,' then, you know, you have to kind of... what I was doing in my code was saying `total_cash =`, you know, I had a data frame, filtering it for that conditional rule, and then, like, summing it off of it. So, like, you have to know your stuff still. You can't just throw, you know—at least not yet—throw a Gemini process and have it just be perfect at it right away. You kind of have to break it down into steps and still code it. But then, you know, you can use LLMs to kind of help check your work, to kind of help you on it if you get in a bind that you can't figure out right away, but you still have to do a lot of the actual logic yourself. And I think, you know, when I was talking about the skills that might change, I do think maybe this—I don't know if this is like bias or wishful thinking—but I do think that accounting will have a moment where it needs to be more programmatic. I'm not saying that we need to become programmers, but, like, I think as a necessary skill set, I think it's going to be important to know the basics of programming, the basics of APIs, you know, of course, how to work with AI, obviously. But I just see our role being much more of, you know, not necessarily actually the actual process of doing something, like the actual manual work, but like, you know, analyzing it more, more helping connect the pieces more with these kinds of automations. So I think having someone who can think critically about what they're doing, break something complicated down into simple steps, and ideally write that out in code or pseudocode, and then work with an engineering team to kind of build that into a more durable automation. I think that's what's going to be valuable in the future because accounting has to be precise. You know, we can't use generative AI everywhere. Like, we again have to be tying things out to the dollars and cents, you know?
Yeah, and if you do that today, you're an extremely valuable employee. So not MVP, but, uh, extremely valuable... EVP? I guess. It took me a second there. So if you're creating these processes, defining them, I think it's a challenge to put yourself in, like, the AI's shoes to take what might seem normal to you and just like you mentioned, like certain specific account numbers are cash, like these assumptions, these contexts, how the business works, how your organization works. If you can distill that and frame the AI in a space that, 'Here's a task, here's how we operate, here's what's important to us, here's what you shouldn't touch.' There's various degrees to which you define the rules—both positive and negative—and prompt techniques and everything. And you go super deep into each of these layers. But at the end of the day, if you can piece together a prototype of something working, you know, that's valuable to you, to your teammates, to the company, to the org, like, you're doing the right thing, and you're positioning yourself to become extremely valuable because that's what companies are looking for. They're looking to adopt AI. They want you to come bring the idea, the project to make it a no-brainer. Like, you know, I think every single company on their balance sheet or in investor calls are just literally talking about AI, but they want to make something happen. If you can be on the ground, you know, distilling these ideas into workflows, into tools, into agents, being that person to push AI forward and be an early adopter and tinkerer, you're going to, you know, do pretty well, and you're going to be ahead of the curve, and once everyone else catches up, you're going to be much, much farther along and become—and probably retain—like the high value that you had when you first started tinkering with AI. So again, something that I try to do in my day-to-day job, but, like, be close to it, try it out. Literally, I think as Ben's describing this, sometimes I think in my head, like, what should I start automating? Because I always think of it, you know, I do like thinking of it, but sometimes you get too in the weeds, you know? You're doing something, you think, 'Oh, this will take an hour,' but maybe if I spent three hours automating it, that one hour might become five minutes in the future. And I think I would challenge everyone listening to the podcast: like, if there's any mundane workflow you're doing next week while you listen to the podcast, just challenge yourself. Say, 'How could I automate this?' AI agents are here, the tools are here, MCP servers are here. How could I distill what I'm thinking, what I'm working with, and make something that's automatable and shareable? Because that is going to be a huge, huge unlock, you know, both at a company, working for yourself. Literally, it's just a workflow and a tool, and you are kind of the wielder of that tool that you create. So definitely very much empower yourself right now.
Yeah, totally. I mean, a lot of, I think, my early automation, like, this is pre-AI, was like, because I just straight up disliked something, I just disliked the process, you know? And there was, like, a natural pain. So, like, yeah, people are listening, and they have some that they just can't stand, you know, that's a great candidate to just be like, 'This, this, let's get this off my plate as much as we can.' And the one thing I didn't touch on too much, because, you know, I went a little bit long on LangGraph, but like, you know, what's great about LangGraph too—and maybe there's going to be a separate follow-on topic, you know, for future podcasts—but like, 'human-in-the-loop,' you know, as kind of a phrase that's coming more and more, you're seeing a lot more with these tools because there's an acknowledgement that, like, AI can't do everything, or AI is great at the actual doing of some work, but the understanding and contextualizing, that sometimes, like, you need a professional, and, like, either just for pure comfort, you know, because you don't trust the code or you don't trust the AI. Or, you know, it's just, you're a professional. You've gone to school. Like, there are some things... like, we don't have AGI yet, you know? Like, we're not there. And so, like, there's still a place for humans to kind of come in and be like, 'OK, let's check that before we proceed to the next step.' So that's what the human-in-the-loop is. And LangGraph makes it really nice and easy to do that because they have kind of checkpoints you can put in the nodes that tell you—or that tell the program, 'OK, stop here and, like, you know, show something,' or 'Stop here and then get an input from the user to say continue.' You know, because that's something that's going to be more and more important too, I think, as people build out their custom, custom automations.
Yeah, I've seen the same thing. Devin, the old, like, AI software engineering agent that came out late last year, kind of took Twitter by storm and made people think software engineering is over. They recently came out with a newer version of Devin that almost exists like Cursor or Windsor for Copilot that is, again, like you mentioned, human-in-the-loop. So it's writing code, trying to generate things, but prompting you, 'Hey, does this look good?' Where the previous version of Devin was hands-off. You described it, it kind of chugged along like an intern, and then came back with a final result. But it was slow, not correct, and didn't give you the kind of control you wanted. So I think, oddly enough, we have better models, but more human-in-the-loop, which, again, seems backwards. But it's people understanding the power of the tools that get 80% of the way there. And with the 20% nudge of an expert or, you know, a domain, you know, kind of owner, then you get that full unlock that I think people were looking for. But from the outset of AI tools, they thought, 'Oh, it's already going to do all this.' But reality check: it's not there yet, but it will be. And I think human-in-the-loop is definitely the basis for what we're using today. And MCP servers and tools are all powered by that, where every time my Personal Capital kind of MCP server wants to go look at some of my data from transactions, it asks me, 'Hey, can I run this tool?' It's like, 'Yes.' Again, if you had a tool that deleted or modified files and you didn't have that ask, I could be deleting files, you know, maybe it shouldn't be deleting. So human-in-the-loop is definitely here to stay. I think probably in the next six months we'll try to reduce that. But today it's definitely something that's top of mind that I don't think many people are pushing stuff to production or having unsupervised agents. It's very, very much supervised.
Alright. So let's do it for bookmarks to kind of wrap it up today. I know we talked about a lot of stuff, so I'll kick it off. My bookmark today is Cursor 0.49. So the new trend of software is not to release a 1.0 ever; you release a 1.0 version after many years. So Cursor is following that rule. They're at 0.49, and there's a Reddit post on the Cursor subreddit saying, 'Oh my God, I basically am using O3, the new OpenAI model, and I'm spending $40 a day in Cursor because there's a new mode that you can basically pay for higher intelligence and it costs per request or per prompt. And I'm, quote-unquote, achieving more than I did with some of my past teams of 10-plus FAANG engineers.' So supposedly it's doing better than engineers at Facebook, Apple, Netflix, Google—the top, quote-unquote, 'top-tier' tech companies. And I think this one went viral because we have new Cursor, new AI IDE, and new models. And together again, like we talked about, the culmination of the tools and the intelligence produces fantastic results. And I myself have tried Cursor with the new Gemini 2.5 Pro Max mode. And we'll talk about it in a different podcast, but it's kind of the same thing: pay for intelligence. And my TL;DR is: it's really damn good and better than it was. I'm not sure it's going to replace 10-plus FAANG engineers. I think that's a clear overshoot. And like we talked about, human-in-the-loop, you're still directing and guiding this thing. No way is it going to be able to do 10-plus autonomous engineers' work. So myth busted, but I think the consensus is there that we're getting to a spot where you can pump out a lot of stuff. And I know Ben was coding a lot this weekend. I'm sure he feels the same that it's, it's pretty damn good. So definitely pick it up.
Yeah. I mean, just like writing long, like, nested for loops, like, having to just not have to do that is so nice. Like, just little things like that. I don't think I can go back to, like, not using it. I think I saw a tweet online that was like, 'I saw a guy on the plane, like, raw-dogging, like, VS Code without, like, Copilot or any AI IDE.' And I thought it was hilarious because I've also found, like, writing code manually just feels weird. I don't know. It's just super odd. But I mean, it's, it's the dopamine hit of, like, just going to the top. Python: you import the libraries at the top. I'm sure other programming languages do the same thing. But like, you know, Cursor, I just write the code and I use those libraries. And then Cursor knows, 'OK, I got to import these at the top.' So it just jumps up to the top and one tab imports, like, 15 lines. It's little things like that are just wonderful.
Yeah, it's great. Cool. So, so my bookmark is actually—so it's a bookmark to a YouTube video that someone on LinkedIn posted. So I'll try and find—I want to give them the credit. Byron Patrick; he's a CPA. I think someone that I was connected with liked his video. And it was really cool because it was actually my first intro into NotebookLM. We mentioned a little bit on this podcast, um, just sparingly. It's probably worth a bigger episode, but it's really cool. It's a Google product. And what's really cool about it is you can give it certain context like PDFs, slides, Google Docs, and then it'll only use that context as, like, its knowledge base. So it won't do any hallucinations. And so it works really nicely again for accountants that gotta be really specific and accurate, that that means a lot. And what was really cool about it is—and what this person posted was—they made a podcast from the context. So this person uploaded a Ripple vs. SEC, like, a court case, which I think is like, I don't know the actual court case. I've heard about it, but there's some lawsuit going on that's kind of famous. Anywho, this person put the court case, you know, these boring legal documents into NotebookLM and then hit a button that says 'generate podcast.' And it generated an AI podcast that was basically two people talking back and forth about, like, the case, sounded super realistic. And it's a great way, especially for someone who, you know, I listen to podcasts, I walk my dog, I, you know, put on a podcast and I go walk and, you know, just kind of nice to have it that way. Um, and so, you know, reading a really boring court case sitting there on paper on my screen, not as exciting, but just being able to listen to it on a podcast is super cool. And so, um, I was tinkering with this at my job, um, you know, for those that don't know, I work at Zillow. And, um, you know, you could give it web pages. So I gave it Zillow's most recent 10-K and generated a podcast out of it. And it talks about, you know, 'Oh, you know, whatever the... whatever we reported in the 10-K.' Right. Um, and it's pretty cool. Like, it's, it's actually, like, pretty, um, pretty awesome. And for those that don't know, a 10-K is like an annual filing that companies do, um, for like financials.
Oh, okay. Well, you know, you never know, you never know.
Alright. Just to be inclusive of everybody. But yeah, so it was really cool. And so I did that for a couple of other, like, more internal documents. And yeah, like it would just... and then I listened to the podcast—you know, 'air quotes' podcast. And it's really good. It's really freaking good.
I did one on a Deep Research query about how to market Split My Expenses. And I think it was like a 17-minute podcast, you know, bickering back and forth with some character. And I was like, 'Darn, I should use this more.' But I hadn't gotten around to using it more, but I really liked my first experience.
Yeah. It's funny. I put something in there that I had kind of written. I put in there like a code—like, it was like a script that did all these things. And the podcast kind of roasted my code a little bit. It was like, 'Oh, this looks a little redundant. What does that mean?' I was like, 'Geez.'
It has a lot of character.
Yeah. Yeah. So it's pretty cool. And yeah, just again, the power of these things. It also has... NotebookLM, it also has, like, mind maps, which are really cool. You can generate FAQs and briefing documents at the push of a button. So yeah, really cool. And again, kudos to Byron Patrick for showing me that because I hadn't even heard of it until I saw that. So it's pretty cool.
Yeah, I think it's a way to be creative, to have boring stuff repackaged into a consumable format. That's kind of the take I have on NotebookLM: make something that you would never ingest or, like, you know, read or listen to, actually entertaining.
Yeah. I mean, just as we wrap up here, thinking about it with your your MCP server for your financials—camera, what it's called? What's the one? Personal Capital.
Yeah. Personal Capital. Like if you could have those transactions and then have it generate a podcast episode. 'Hey, this is what you spent this week.' Yeah. You know, like, 'Hey,' just that way you can hear it, because sometimes it's nicer just to read it. You know, 'Hey, Bradley, cool it down. Chipotle too many times.'
Yeah, yeah. I'd have a McDonald's... uh, I get the Diet Cokes like every day. So yeah, it'd be probably pretty brutal. But yeah, Google, if you're listening, please put an API on that. We would love to use it and make it as cheap as your Gemini models, because those are at a nice, great price point for us developers.
Yeah, yeah, pretty cool stuff. Cool. Awesome. Well, I think that'll wrap it up. Uh, good stuff, Brad, as always. And, um, yeah, until next time.
Until next time. Thank you for listening to the Break Even Brothers podcast. If you enjoyed the episode, please leave us a five-star review on Spotify, Apple Podcasts, or wherever else you may be listening from. Also, be sure to subscribe to our show and YouTube channel so you never miss an episode. Thanks. Take care.
All views and opinions by Bradley and Bennett are solely their own and unaffiliated with any external parties.
Creators and Guests
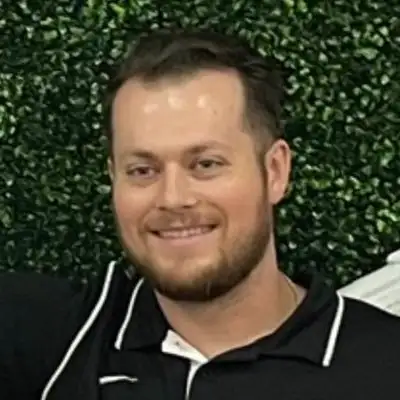
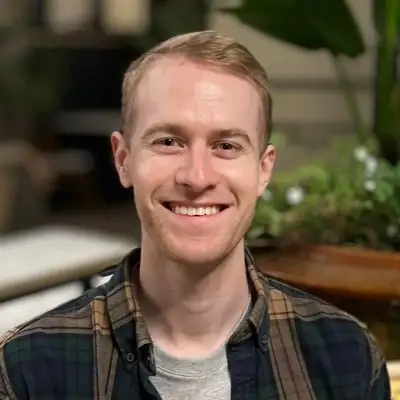
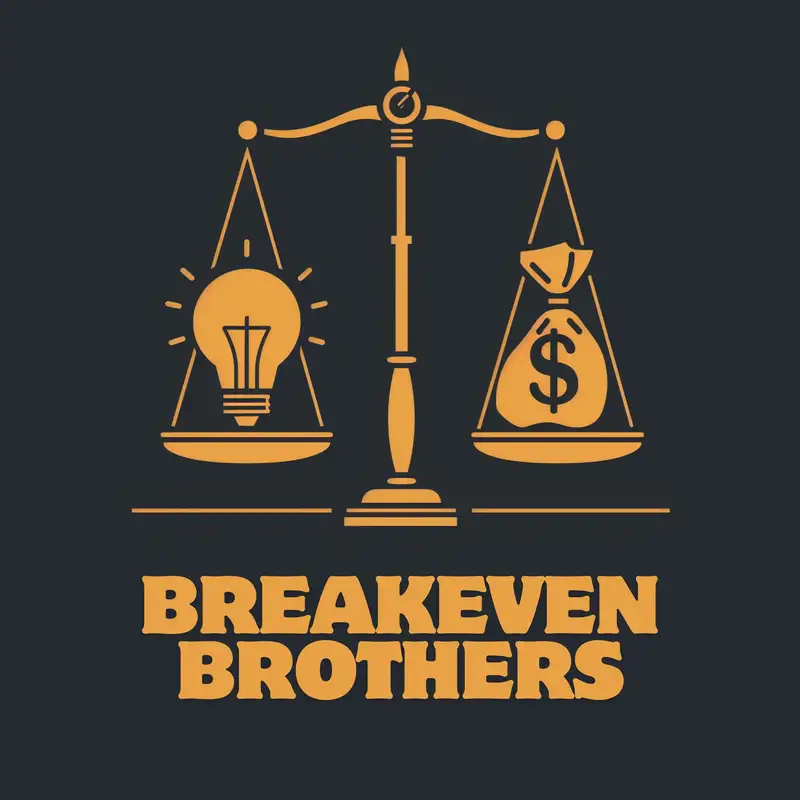